Advancing data center networking through open access
2022๋ 10์ 27์ผ
์ ์: Ian Evans
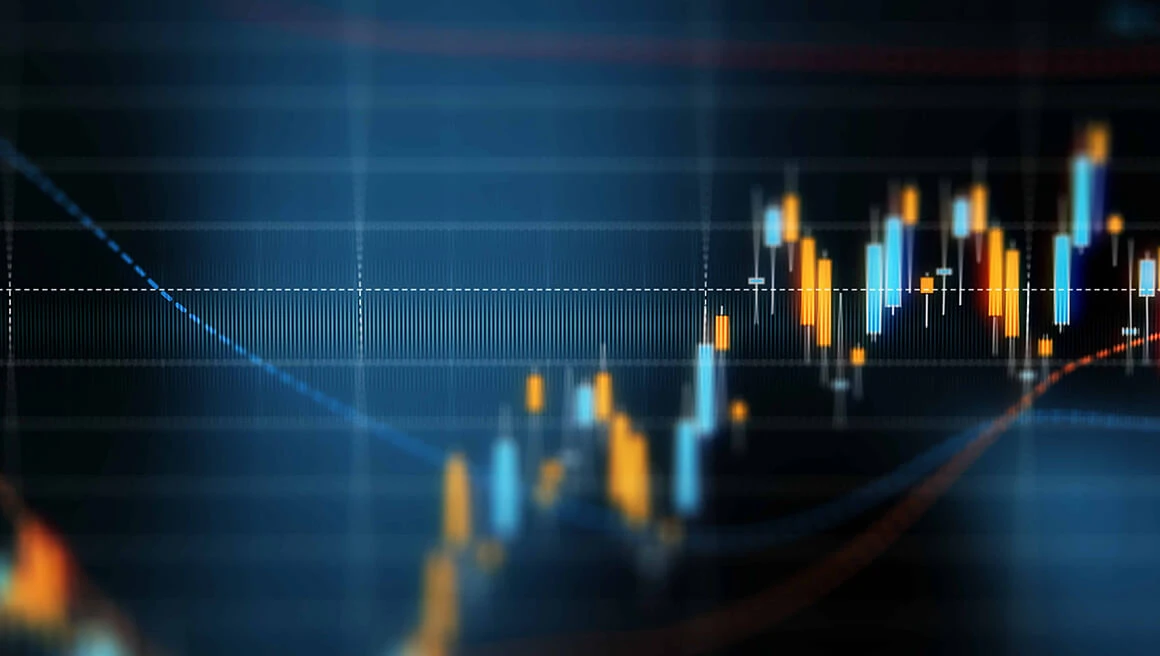
A team from UCL creates an open access tool to benchmark and investigate data network systems
In the image above: Christopher Parsonson is a PhD candidate in AI & Networks at University College London (UCL). As part of a team lead by Dr Georgios Zervas, Associate Professor of Optical Networked Systems, he produced an open access framework called TrafPy, which was recently published in the Elsevier journalย Optical Switching and Networking. Whenย Christopher Parsonsonย ์ ํญ/์ฐฝ์์ ์ด๊ธฐ, a PhD candidate in AI & Networks at UCL, was working on research on optical data center networks, the team he was part of knew they wanted to publish their work in an open access journal. Led by Drย Georgios Zervasย ์ ํญ/์ฐฝ์์ ์ด๊ธฐ, Professor of Optical Networked Systems at UCL, they set out to create an OA tool in an area that would have a massive impact on large-scale artificial intelligence models โ and therefore much of modern living. Chris explained:
We realized there was a lack of open access research around data center networks in general, but in particular the starting point for everything in this area is being able to benchmark different systems. There wasnโt an open access tool for doing that. We knew that creating one was key to accelerating progress, validating new systems, and making everything reproducible.
As part of Prof Zervasโs team, Chris led a project to produce anย open access framework called TrafPyย ์ ํญ/์ฐฝ์์ ์ด๊ธฐ. Their paper was recently published in the Elsevier journalย Optical Switching and Networkingย ์ ํญ/์ฐฝ์์ ์ด๊ธฐ, Compatible with any simulation, emulation or experimentation environment, it can be used for standardized benchmarking and for investigating the properties and limitations of network systems. It provides a way to benchmark systems against those developed by other research teams โ a crucial element for understanding whether progress is being made.

Prof Georgio Zervas, Ph.D
One of the reasons optical data center networks are so important is that they open the door to a large number of emerging applications, from AI models to genome processing systems. They have the potential to accelerate research in everything from health and technology to entertainment.
โJust recently we were hearing aboutย BigScienceโs new large language modelย ์ ํญ/์ฐฝ์์ ์ด๊ธฐ,ย BLOOMย ์ ํญ/์ฐฝ์์ ์ด๊ธฐ, which can generate text in 46 different languages and 13 programming languages,โ Chris said. โThatโs made up of 176 billion parameters.โ
To train a model of that scale, researchers use multiple computers. In the case above, the model is distributed across 1,024 different devices.
โThat requires communication across all these devices, which increases the more devices you use,โ Chris explained. โThat means that the bottleneck is no longer about individual computers but about the network that connects them. Over the last 18 years, thereโs been an 8-factor decrease in the number of bytes communicated per flop.
โIn other words, the performance of computers is increasing much faster than the rate at which weโre increasing the performance of our data center networks.โ
The source of that limitation is that current data centers use electronic switching, meaning they have poor scalability, low bandwidth and high latency. Whatโs more, theyโre energy intensive. Data centers and data transmission networksย each account for about 1% of the worldโs energy consumption, predicted to rise to 10% to 15% of total energy consumed by 2030. In some cases, Chris said, more than 50% of that power consumption is from the network performing communication tasks between devices:
If we want to scale to next generation applications with brain-scale neural network models, which might have 100 trillion parameters, we need next generation data center networks where the interconnects are all optical. They have much lower latency, take up less space and โ because they are passive devices that donโt need cooling โ use considerably less power.
The team Chris works on is already working with industry giants such as Microsoft on applications for the technology, and theyโre working with people in multiple fields. Because data analysis underpins such a vast range of disciplines, Chris finds himself in conversation with people from a diverse range of areas, from physics to electrical engineering and computer science. That broad reach was one of the reasons they were keen to publish open access:
The more you publish open access, the more likely it is that people in different fields are going to find your work, see the findings youโve published and apply them in their own research.
Chris noted that the area he works in has lagged behind computer science in terms of research it publishes open access. One of the teamโs goals was to redress that balance:
Computer science has a strong culture of open access, and over the past 10 years, itโs clear that that has been a big driver of progress in areas such as machine learning.
Chris pointed toย AlexNetย ์ ํญ/์ฐฝ์์ ์ด๊ธฐ, an open-source neural network tool for image recognition that competed inย the ImageNet 2012 Challengeย ์ ํญ/์ฐฝ์์ ์ด๊ธฐ. As Chris puts it, AlexNet โabsolutely smashedโ the other competitors:
That spurred the next decade of interest and investment in machine learning and turned it into one of the biggest fields of research.ย
โIn data center networking, you havenโt seen so much of that development of open access benchmarks, simulations and systems,โ he continued. โEven when research is published, the researchers often hold back from sharing the back-end algorithms or datasets they were using, so itโs hard to reproduce the results, to test against old systems and develop new ideas.
โOur work is to try and redress the balance and tackle some of the shortcomings in open access in data center networking.โ
With open access as a must, Chris was also looking for a platform that would bring his work to the people who could use it, which was one of his reasons for choosing to publish with Elsevier.
Obviously Elsevier โ throughย ScienceDirectย โ has a really big audience. And Optical Switching and Networking is a journal that caters to the kind of application areas weโre interested in โ next-generation optical data networking centers. They had the open access option, a swift review process and high-quality papers that seemed to get a lot of traction. That was very appealing to us because we needed to make sure that wherever we publish, people end up seeing the work and using the tools that weโve developed.
Of course, to maintain the high quality of papers a journal likeย Optical Networkingย has to have a rigorous peer review process. For some, that can be a slightly nerve-wracking or even frustrating process, but Chris was impressed with the speed and quality of feedback:
Iโve published in a few places recently, and this was extremely, extremely good. Usually, the slightly painful thing about the review process is the length of time it takes to get things done. Here, we submitted and then two-and-a-half months later, we had the reviews back, which is really good.
We changed the paper following that feedback, and then I think a day later it was approved and was then immediately available online for people to read. Thatโs much faster than my previous experience.
Chris also found the process useful in improving the paper:
Throughout the process, we were in communication with the editors, and they were very helpful in helping us realize how to frame our work. That really helped accelerate the review process.
Now that the work is published and available, Chris and the team see it as potentially providing the same kind of boost for data center research as ImageNet did for machine learning in 2012:
Our hope is that TrafPy will be used as the foundation with which to establish rigorous benchmarks and facilitate the next decade of development in data center networks. Weโve made it completely open-source, so anyone can download it and use it any way they wish. That is the key to accelerating progress.
๊ธฐ์ฌ์

IE